solving the problem of overfitting:regularization
- 发生的在linear regression上面的overfitting问题
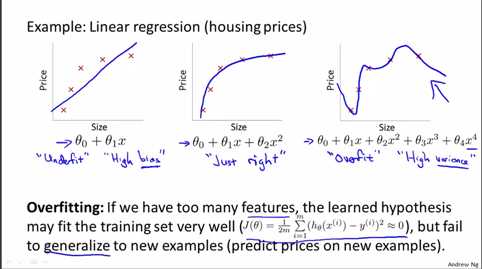
- 发生在logistic regression上面的overfitting
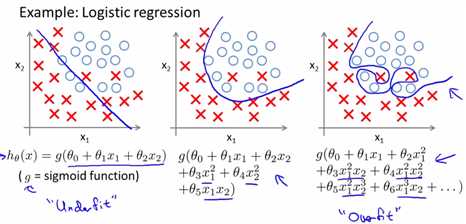
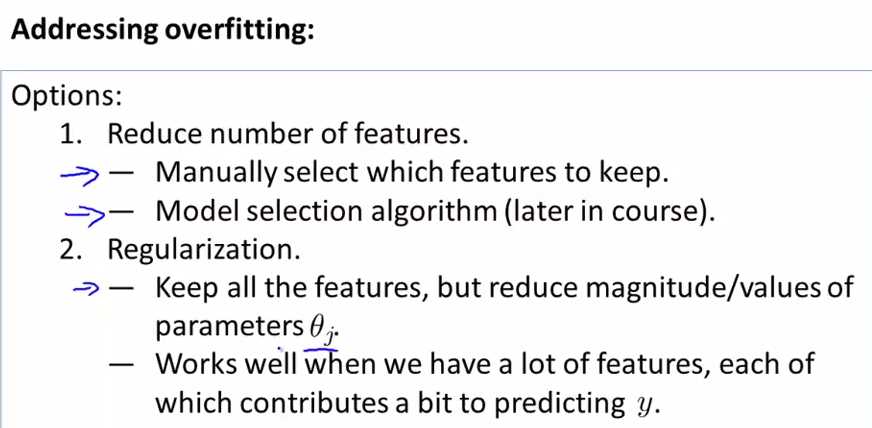
- regularization: cost function of linear regression
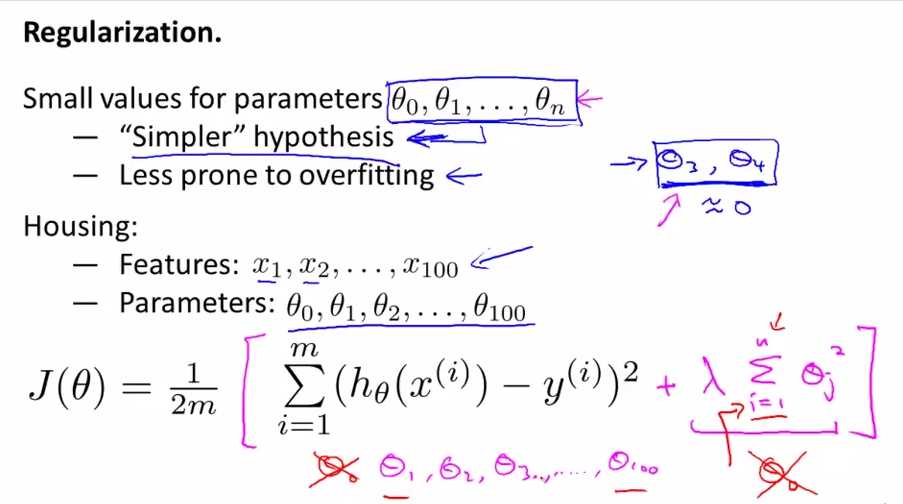
-
- parameters小的话,这样hypothesis就会变得简单,这样就不会overfitting
- 一般不会对θ0进行regularization
- 上式是进行regularization的linear regression的cost function,要使上式的值取最小值
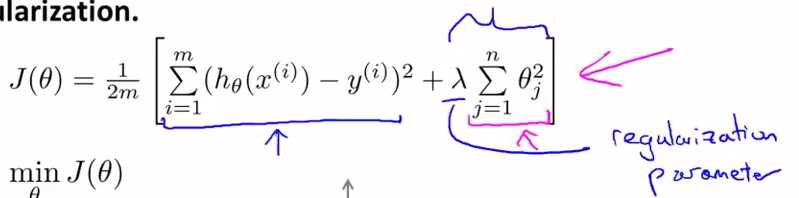
-
- 由两个式子(两个目标)组成,第一个式子是为了对trainning data更好的拟合(fitting the training data),第二个式子是为了避免overfitting
- 第二个式子叫regularization term, λ叫regularization parameter, λ是为了平衡两个目标用的
- 如果 λ非常大的话(这时θ1-θn几乎为0,hypothesis变得很简单,只有常数),就会出现underfitting,对trainning data很低的fitting
machine learning(12) -- solving the problem of overfitting:regularization
原文:http://www.cnblogs.com/yan2015/p/4844930.html